In the information economy, data is a thriving currency. Every company is looking to collect the massive influx of raw material that forms their information collection and analysis processes. However, decontextualised numbers and facts are everywhere with little rhyme or reason. This is where data mining comes in.
Data mining is a set of processes that enables companies to analyse large databases as a means of generating actionable data insights. While basic data mining tasks can vary across different departments, the entire field is useful for many sectors of business. This burgeoning technology has also seen major growth outside of firms that implement it for their uses, with consultants offering it as a service.
As a natural extension of the demands of the information age, its development as a tool has expanded in recent years. It has proliferated outside of business, opening up fields like scientific data mining for research purposes. Let’s have a look at what it offers.
Data Mining for Business Analytics
Data mining processes can be very useful for a range of different business activities. However, one must first understand that data mining, as a concept, has multiple techniques within it. Here are some of the main categories and how businesses implement them.
- Classification: As the name suggests, this is the process of taking data and giving it distinct categories for further use. For example, a fashion retailer might sort their products into shirts, T-shirts, underwear etc. This helps them learn more about each buyer.
- Clustering: Clustering is similar to the previous technique, but the categories are more general. Retail stores could cluster their data into men’s and women’s clothes for example. This is followed by factor and cluster analysis.
- Association Rules: tracking patterns based on linked variables. For supermarkets, this would be like someone buying 2 food items that go together, e.g. macaroni and cheese. The store will know what to do with these items, put them closer, and put them on sale together etc.
- Regression Analysis: this aids in statistical analysis and data mining practices by identifying variables that occur in conjunction. It helps pinpoint probabilities, determining the relationships between 2 variables. Example: if there is an increase in demand for good A, then there will be an increase in demand for good B.
- Anomaly or Outlier Detection: This one looks into the odd dataset among the norm. It’s not enough to identify a trend, but one must also investigate the average of most trends. It studies outliers and understands what caused them. For example, there is a massive increase in men shopping for sweets normally bought by women. One can wrongly infer that it was a result of a shifting permanent trend or they can recognise that it’s February and Valentine’s Day is around the corner.
Data Mining vs. Data Analysis
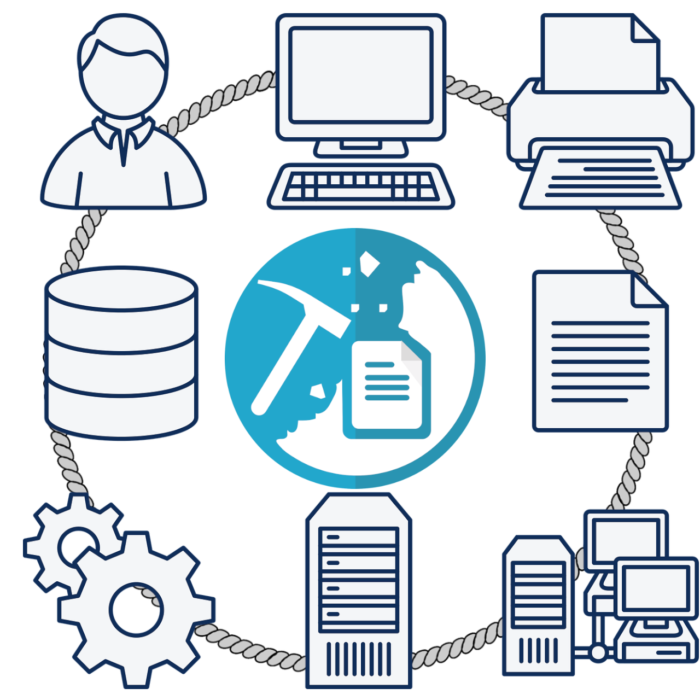
While data mining is used in data analytics, they are not interchangeable terms. Data mining is a step before this. It’s all about using a framework to make data usable. It is the process of taking massive swathes of raw points of data and giving them a structure. Data analytics has rules and patterns that it follows, whereas mining does not (initially) have such rules or pre-existing patterns.
Take social network data mining vs. social media data analysis for example. In the former, data gathering happens en masse. Data is often stored in or retrieved from advanced data warehousing operations.
Business Intelligence & Predictive Analytics
While predictive web analytics is all the rage these days, companies can one-up these efforts with mining. For example, a business could create better online retail data analytics by looking through catalogues of purchase information from a variety of stores. This information can be categorised to create a predictive data set for online targeting and customer segmentation purposes.
Using predictive data analytics for customer acquisition is nothing new. Institutions like the University of Sydney have been employing them for a range of purposes. It has aided in enrolment procedures and even in identifying students with high chances of providing endowments to the school. Conversely, an analysis may use research that is pre-categorised from Google or Facebook or on-page analytics.
Some of the characteristics of data mining that set it apart from analytics are:
- Massive quantities of uncategorised data.
- Data structures are so complex that conventional statistical analysis is not possible
- The data is often noisy and incomplete.
- Data mining analyses are either predictive or descriptive.
Examples of Data Mining in Business
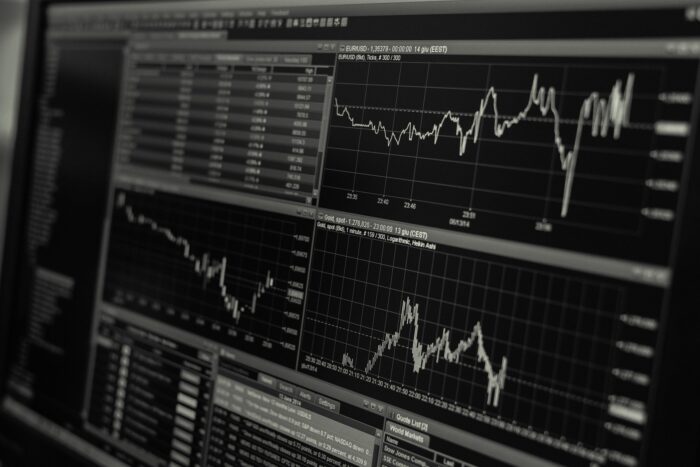
There are many applications of these processes. Data mining and segmentation techniques have long been employed by dozens of companies. Studies have outlined their applications for multi-segment marketing and retail. Similarly, big data customer insights often rely on many of the methods listed here. Most famously, companies like Cambridge Analytica used it for controversial election campaigning.
Cluster analysis marketing often relies on this sort of data and without the categorisation that mining provides, it can often be very difficult. Together, these processes form a range of advanced market segmentation practices on the consumer side to find more buyers. It can even have predictive abilities in terms of customer acquisition data analytics and finding desirable behaviours.
Mining procedures can also aid in giving form to unstructured stats from rapid data point retrieval. Real-time data collection can put a lot of numbers in your hands. However, making sense of a chunk of it can refine the process as it happens.
In the same vein, creating clusters for data you receive via social media can then help you track more of similar data if need be or allow for predictive analyses. This can be preferable to receiving random data if you’re looking for specific variables, target groups, or behaviours.
Business intelligence testing is another way to make use of these methods. Data mining is the first step to testing hypotheses about your competition and setting up “war games” to define best practices against competitor activity or creating effective buyer personas.
Data Collection Solutions
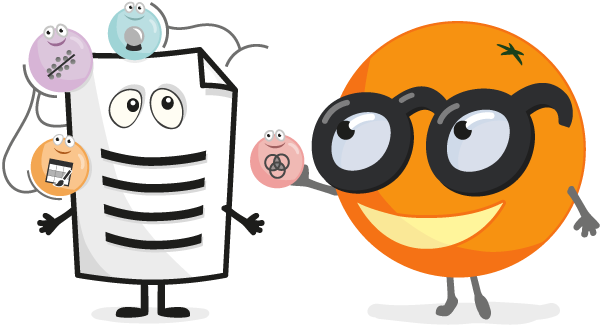
Here are some programs for data processing and a brief summary of which areas they excel at:
DataMelt is useful for crunching numbers. It offers programs for mathematics, statistics, calculations, data analysis, and visualisation. ELKI framework, meanwhile, focuses more on algorithms, offering great systems for cluster analysis. ELKI is also more user-friendly for researchers, students, and business organisations.
Orange data mining helps organizations do simple data analysis and use top visualization and graphics. Great for creating heat maps, hierarchical clustering, decision trees. Rattle GUI works on statistical and visual summaries of data, prepares it for modelling, and then utilises machine learning operations to present the information.